The R&D 100 awards are much like the Oscars, but for researchers: It’s an honor just to be nominated as a finalist. Two teams from UT recently had that honor, with one team also named a winner.
A team from the Center for Ultra-Wide-Area Resilient Electric Energy Transmission Networks (CURENT) was chosen as a winner of a R&D 100 Award in the Software/Services category for their Large-scale Test Bed (LTB). Another team from the Min H. Kao Department of Electrical Engineering and Computer Science (EECS) was honored as a R&D 100 Finalist in that same category for their Deep Convolutional Neural Network for N-1 (DCNNN).
Helping Understand the Grid of Tomorrow
The LTB was designed to mimic power grids of the future, allowing researchers to design, implement, and test new technology and modelling related to the grid, particularly when integrating renewable energy.

James W. McConnell Professor Fran Li
“It is a significant technology advancement from existing power system simulations,” said EECS James W. McConnell Professor Fran Li, who also serves as CURENT campus director for UT and the LTB project lead. “I wholeheartedly thank our team members for their tremendous effort to make this happen, especially EECS Research Assistant Professor Hantao Cui, my former doctoral student, for his exceptional contributions to the research and development of LTB.”
The LTB is set up to run simulations based off of a wide variety of factors, allowing insight into what reactions to particular incidents might be as well as new methods of control.
More Rapidly Securing the Grid
DCNNN is a collaboration among Li, Old Dominion University, and the northeast power authority ISO-New England. It aims to improve power grid security at speeds more than 100 times faster than traditional methods by employing fully-connected, smart neural networks.
“DCNNN allows us to have contingency screening and cascading failure assessment with high accuracy, and is partially funded by a previous NSF project of mine” said Li, who also led the DCNNN project. “I’d like to specifically thank my former doctorate student, Yan Du, for developing the algorithms using deep learning, which was a completely new area for her when we started the work three years ago.”
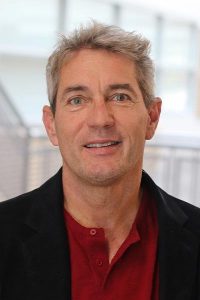
CTI Professor Kevin Tomsovic
Li also noted the importance of the leadership provided by CURENT Director Kevin Tomsovic, who is also CTI Professor in EECS and a co-Lead of the CURENT LTB project, pointing out that this year’s R&D 100 honors are just the latest successes that the center and the department have had.
The R&D 100 awards have been given annually for almost 60 years. Judges from around the world go through submissions before coming up with a list of finalists, ultimately narrowing those lists down to a varying number of winners in each of six categories.
The annual conference is a major event in the scientific community, but, like many other aspects of life, was put on hold due to COVID-19. This year, R&D World Magazine held a virtual conference, announcing finalists one week before revealing winners online over a period of three days in October.